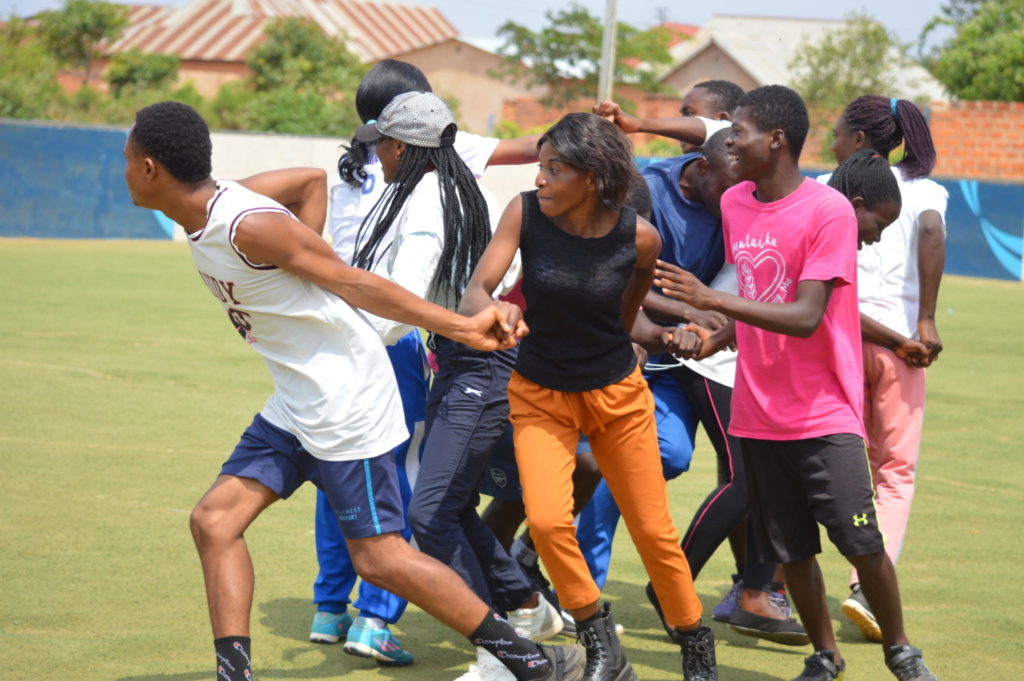
By Ojo Emmanuel Ademola – In the rapidly evolving landscape of artificial intelligence (AI), issues of fairness and equity are becoming increasingly paramount. As these technologies take on larger roles in everything from hiring practices to healthcare, it is crucial to understand how biases embedded in data can perpetuate and even exacerbate existing inequities. One of the most pressing concerns is the entrenched gender disparities, particularly those that intersect with racial biases. Focusing on *women of colour*, this discussion navigates the intricate dynamics of bias in data and AI, shedding light on the multifaceted nature of these disparities and exploring potential solutions for a more equitable future.
Definitively, unveiling Bias with an attempt to navigate Gender Disparities in Data and AI involves a critical examination of the intrinsic and extrinsic factors that perpetuate gender inequality within the realm of artificial intelligence. Issues such as biased algorithms, the underrepresentation of women in AI fields, and the lack of diverse data sets illustrate the systemic nature of these disparities. Thought processes integral to addressing these challenges encompass both technical and ethical considerations, including the necessity of incorporating diverse perspectives during the development phase and fostering an inclusive culture within tech industries. Potential solutions to mitigate gender disparities range from implementing comprehensive bias detection and mitigation strategies to promoting and supporting the education and career advancement of women in STEM. Moreover, developing policies that enforce transparency and accountability in AI systems can ensure that biases are identified and rectified. Collectively, these efforts pave the way for a more equitable and fair AI landscape that benefits all members of society.
Let’s excavate this intriguing discussion with such a leaning on addressing this bias to showcase a thorough understanding of its origins, manifestations, and potential solutions.
*Issues*
1. *Historical Data Bias*: Historical data often reflect the societal biases of the period in which they were collected. If such biased data are used to train AI models, the resultant systems will perpetuate existing inequalities. For instance, if a dataset regarding employment is tainted by historical gender discrimination, an AI-driven hiring platform trained on such data may continue to favour male applicants over female ones.
2. *Underrepresentation*: Women and gender minorities are frequently underrepresented in datasets. This underrepresentation can lead to algorithmic models that do not work as accurately for these groups. A notorious example includes facial recognition systems that perform significantly worse for women or individuals with darker skin tones.
3. *Algorithmic Bias*: Even when data seems neutral, the algorithm’s design can introduce bias. For example, if a predictive policing algorithm is trained on biased crime data, it might disproportionately target communities where women and non-binary individuals could already be vulnerable.
4. *Feedback Loops*: Bias in AI systems can create and reinforce feedback loops, wherein biased outputs feedback into the system, further entrenching and amplifying disparities. For instance, biased AI systems in hiring might reduce the number of women entering tech fields, which in turn reduces female representation in future data.
*Thought Processes*
1. *Awareness and Acknowledgment*: Recognize and acknowledge that data and algorithms are not neutral. Each step of the data pipeline (collection, cleaning, analysis, and implementation) carries the potential for bias.
2. *Inclusive Data Collection*: Strive for inclusivity in data collection by ensuring that datasets represent diverse populations. This includes gender, but also ethnicity, socio-economic status, and other factors.
3. *Bias Detection and Measurement*: Develop and utilize methodologies to detect and measure bias in datasets and algorithms. Tools such as fairness metrics and auditing frameworks can assist in identifying disparities.
4. *Intersectional Approaches*: Employ an intersectional lens to understand how overlapping identities (such as gender, race, and class) may influence how individuals are affected by AI systems. Intersectionality can reveal the compounded disadvantages facing marginalized groups.
*Solutions*
1. *Diverse Team Composition*: Encourage diverse representation among teams developing AI systems. A variety of perspectives can help identify and mitigate bias at different stages of AI development.
2. *Ethical AI Frameworks*: Adopt ethical AI frameworks that prioritize fairness, accountability, and transparency. Frameworks like these can guide the responsible development and deployment of AI technologies.
3. *Bias Mitigation Techniques*: Implement bias mitigation techniques such as re-weighting training data, using fairness constraints in algorithms, and post-processing model outputs to correct bias.
4. *Regular Audits and Impact Assessments*: Conduct regular audits of AI systems to monitor for bias. Impact assessments can also help understand the real-world implications of AI systems and ensure they do not adversely affect marginalized groups.
5. *Policy and Regulation*: Advocate for and adhere to policies and regulations designed to promote fairness in AI. Governments and regulatory bodies can play a crucial role in setting standards and ensuring compliance.
6. *Continuous Learning and Training*: Provide continuous education and training for AI practitioners on topics of ethics, fairness, and bias in AI. Equipping teams with the knowledge and tools to address bias is crucial for long-term change.
7. *Community Engagement*: Engage with affected communities to understand their concerns and experiences with AI systems. Feedback from diverse groups can provide critical insights and foster trust in AI technologies.
Centrally, Navigating Gender Disparities in Data and AI involves a critical examination of the intrinsic and extrinsic factors that perpetuate gender inequality within the realm of artificial intelligence. For instance, consider the experiences of women of colour in the tech industry—issues such as biased algorithms and underrepresentation create a compounded disadvantage that reflects wider societal biases. The lack of diverse data sets often results in AI systems that fail to recognize or accurately respond to the needs of these women, further entrenching existing disparities. Addressing these challenges requires a thought process that integrates both technical and ethical considerations, such as incorporating perspectives from women of colour during the development phase and fostering an inclusive culture within tech industries. Solutions may range from implementing comprehensive bias detection and mitigation strategies to promoting education and career advancement opportunities specifically for women of colour in STEM. Moreover, developing policies that enforce transparency and accountability in AI systems is crucial for ensuring biases are identified and rectified, paving the way for a more equitable and fair AI landscape that benefits all demographics.
Surmising, the gender disparities in data and AI, particularly for women of colour, demands a nuanced and comprehensive approach. By understanding and mitigating the unique intersectional biases faced by this demographic, we can develop AI systems that promote fairness and equality. Incorporating diverse perspectives, adopting inclusive practices, and engaging with affected communities are essential steps toward achieving these goals.
Conclusively, addressing the gender disparities in data and AI, particularly for women of colour, is not merely a technological challenge but a profound moral imperative. By acknowledging the compounded biases these individuals face, and by making concerted efforts to collect inclusive data, employ diverse teams, and implement robust ethical frameworks, we can work towards AI systems that are genuinely fair and equitable. This path forward requires a collaborative approach—engaging communities, advocating for informed policy changes, and continuously educating AI practitioners. Only by recognising and actively mitigating the unique intersectional biases can we ensure that these powerful technologies serve to uplift all segments of society, driving progress towards a more inclusive and just world.